Pocket brains: Neuromorphic hardware arrives for our brain-inspired algorithms
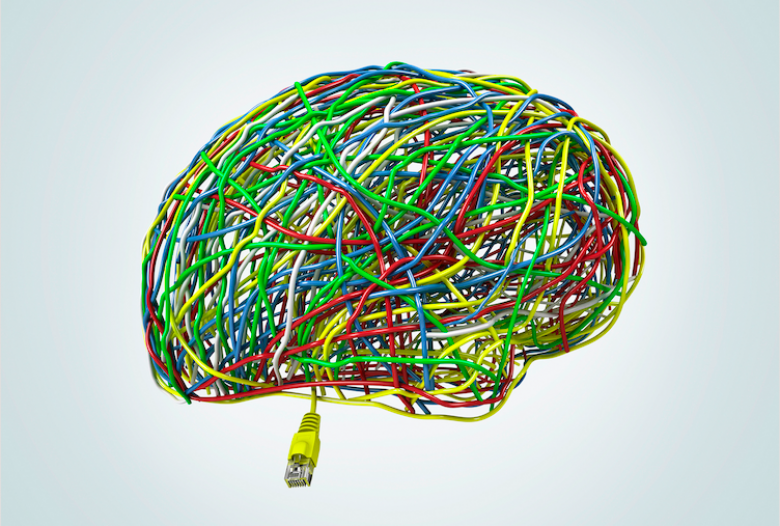
As the world’s great companies pursue autonomous cars, they’re essentially spending billions of dollars to get machines to do what your average two-year-old can do without thinking—identify what they see. Of course, in some regards toddlers still have the advantage. Infamously last year, a driver died while in a Tesla sedan—he wasn't paying attention when the vehicle's camera mistook a nearby truck for the sky.
The degree to which these companies have had success so far is because of a long-dormant form of computation that models certain aspects of the brain. However, this form of computation pushes current hardware to its limits, since modern computers operate very differently from the gray matter in our heads. So, as programmers create “neural network” software to run on regular computer chips, engineers are also designing “neuromorphic” hardware that can imitate the brain more efficiently. Sadly, one type of neural net that has become the standard in image recognition and other tasks, something called a convolutional neural net, or CNN, has resisted replication in neuromorphic hardware.
That is, until recently.